Deepfake face detection
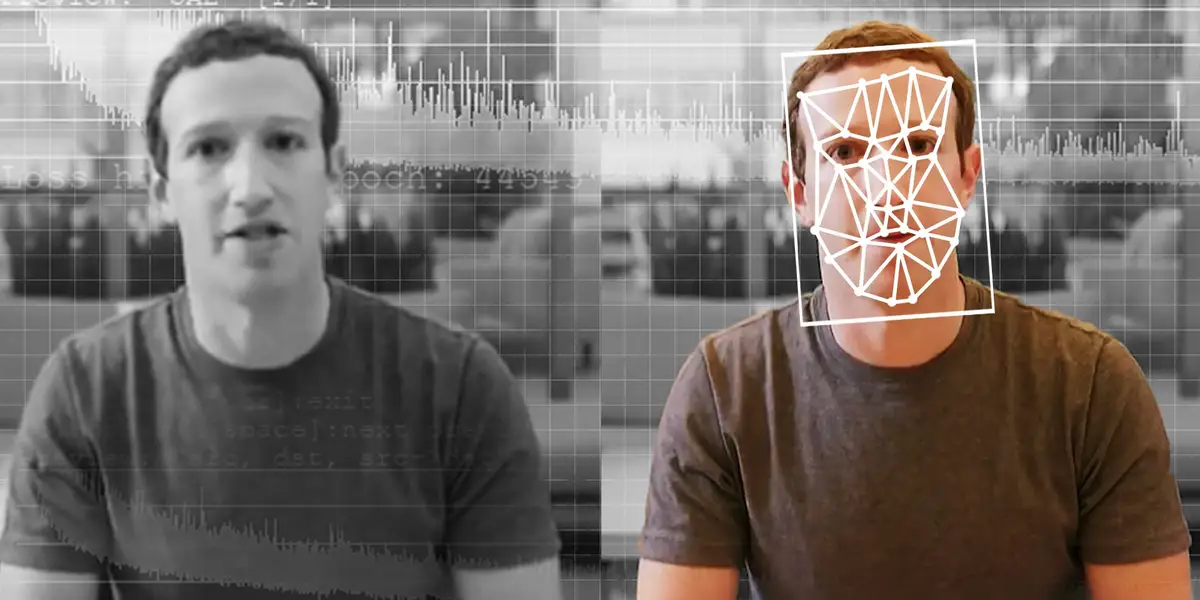
This project develops a deep fake face detection system using a novel pairwise learning model to detect face images generated by various GAN architectures. The model architecture includes the Common Fake Feature Network (CFFN) for extracting discriminative features and the Classification Network (CN) for classifying real or fake images.
The training process involves minimizing the L2 norm distance between genuine and fake pairs. The model was evaluated using precision and recall metrics with the CelebA dataset and demonstrated improved precision and generalizability after retraining with an expanded GAN dataset, using Fréchet Inception Distance (FID) and Inception Score (IS) metrics.
The project successfully outperformed existing models, showing potential for future work on different loss functions, higher-quality fake face datasets, and subclassification based on GAN models.
View this project on GitHub: link